MONET Summer School - May 15-19 2000, Bertinoro, Italy
The MONET
summer school on Model-Based Systems and Qualitative Reasoning (MBS/QR)
was held at the University Residential Centre of Bertinoro (Italy) on
May 15-19, 2000 (Monday 09:00 until Friday 17:00).
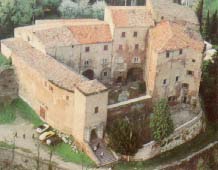 |
|
The
Summer School took place in the University
Residential Centre of Bertinoro. The Centre, built inside the
age old Stronghold and its fortified walls, has been operating
since June 1994 as the seat of University training courses, of
national and international meetings and congresses as well as
qualifying cultural events.
Further
details of location
|
Introduction
The goals of the Summer School were:
- To inspire and prepare the next generation of researchers in the
field of MBS/QR.
- To convey to young industrialists the potential of MBS/QR
technology for solving problems resulting from interacting with
complex systems and their behaviour.
- To communicate the type of problems that can be solved by applying
MBS/QR technology.
- To present a coherent and comprehensive overview of the main
results and achievements of MBS/QR research.
- To layout the state of the art of MBS/QR technology, highlight
problems and open issues, and point out the research opportunities for
the near future.
Back
to Top
Lecture Plan (Subject to minor changes)
|
Monday 15 |
Tuesday 16
|
Wednesday 17
|
Thursday 18
|
Friday 19
|
Morning |
Welcome |
|
|
|
|
|
A1 |
A3 |
A4 |
A5 |
B5 |
|
|
|
|
|
B6
(unit 1) |
|
|
|
|
|
|
Afternoon |
A2 |
B1 |
Soc. |
B3 |
B6
(unit 2) |
|
|
B2 |
|
B4 |
B7 |
|
|
|
|
|
Closing |
Back
to Top
Lectures, Units and Topics (Subject to minor
changes)
Three hour
lectures
A three hour lecture consisted of 3 units.
Each unit lasts one hour, including a 15 min break between units.
Lecture A1: Introduction to Qualitative Modelling and
Simulation
(Teacher: Ken Forbus )
- unit A1.1: motivation
- problems, principles, ideas and theories/approaches
- what is the role of simulation (prediction/postdiction)
- QR/MBS history
- unit A1.2: qualitative model(ling)(s)
- ontologies for simulation models
(components, processes, constraints, functional models, etc.)
- quantities, qualitative values and quantity spaces
- dependencies
(influences, proportionalities, inequalities, etc).
- basic concept of qualitative calculus
(incl. the notion of ambiguity)
- unit A1.3: model construction and simulation
- model fragments
- qualitative states, state transition and simulation
- properties: completeness, soundness
Back
to Top
Lecture A2: Advanced Topics in MBS/QR
(Teacher:
Unit A2.1 & A2.3 Ken
Forbus and Unit A2.2 Peter
Struss)
- unit A2.1: causality
- causality (what is meant by it?)
- causality as a basis for prediction and explanation
- techniques for causal ordering
- unit A2.2: advanced qualitative calculus
- qualitative calculus
- order of magnitude reasoning
- interval reasoning
- unit A2.3: modelling paradigms
- compositional modelling
- abstractions (time scale, parameter)
- points of view (behavioral, functional, etc.)
Back
to Top
Lecture A3: Model-Based Diagnosis
(Teacher: Luca Console)
- unit A3.1: introduction and motivation
- overview of diagnostic approaches / problems
(rule-based, functional, causal models etc., should lead to
the conclusion: MBD is a specific type of diagnosis)
- why model-based ?
(what are the special features, why is it better)
- component-connection model
- consistency-based diagnosis
(basic steps including: detecting discrepancies, conflict
generation, candidate generation, and probing)
- unit A3.2: multiple and large scale models
- fault models
(including elaboration on: consistency versus abductive
diagnosis)
- multiple (fault) models, structural models, hierarchical models
dealing with large scale models
- unit A3.3: examples, non-technical domains and remaining
problems
- applications and examples
- MBD in non-engineering/technical domains
- remaining problems and future research goals
(e.g. bridge-faults, time varying faults)
Back
to Top
Lecture A4: Qualitative Reasoning and Educational Systems
(Teacher: Bert Bredeweg)
- unit A4.1: introduction and motivation
- overview on research on cognition and learning (brief)
(highlight notions such as: novice/expert distinction, common
sense reasoning, analogical reasoning)
- why use simulation models?
(what are the benefits, how are they used, methods of
teaching)
- relevance of articulate models (in ILE)
- unit A4.2: teaching functions in Interactive Learning
Environments (ILE)
- diagnosing learner behaviour
- generating explanations
- visualisation of simulation models/results
- model progression
- unit A4.3: examples and remaining problems
- CSCL and other WWW related issues
- applications and examples
- remaining problems and future research goals
Back
to Top
Lecture A5: Qualitative and Semi-Quantitative Simulation
(Teacher: Ben
Kuipers)
- unit A5.1: modeling and simulation with incomplete knowledge
- introduction and motivation
- Qualitative Differential Equations and qualitative behavior:
- Qualitative Simulation
(incl. constraint filtering, behavior filters, behavior
abstraction)
- research problem/question
- unit A5.2: semi-quantitative simulation
- partial quantitative knowledge, semi-quantitative differential
equations
- semi-quantitative simulation: Q2, Q3, NSIM
- semi-quantitative system identification
- open research problems
- unit A5.3:
- abstraction methods that help make qualitative simulation
tractable
- temporal logic methods that make it possible to query the
results of qualitative simulation automatically (rather than
inspecting them by hand)
Two hour lectures
A two hour lecture
consists of 2 units. Each unit lasts for one hour, including a 15 min
break between units.
Lecture B1: Model-based Diagnosis in the Automotive Industry
(Teacher: Peter
Struss)
- unit B1.1: introduction, motivation and problems
- what are the characteristics of the task and/or domain
- what are the problems (in terms of the domain and/or task)
- what are the challenges for the MBS/QR technology
- problems and solutions
- unit B1.2: examples and remaining problems
- problems and solutions (cont'd)
- applications and examples
- future (research) goals
Back
to Top
Lecture B2: Model-based Reasoning in Ecology Domains
(Teacher: Peter
Struss)
- unit B2.1: introduction, motivation and problems
- what are the characteristics of the task and/or domain
- what are the problems (in terms of the domain and/or task)
- what are the challenges for the MBS/QR technology
- problems and solutions
- unit B2.2: examples and remaining problems
- problems and solutions (cont'd)
- applications and examples
- future (research) goals
Back
to Top
Lecture B3: Model-based Reasoning for Control tasks & Medical
applications
(Teacher: Unit B3.1 Ben Kuipers and Unit
B3.2 Liliana Ironi)
- unit B3.1 Model-based reasoning for control tasks
- introduction
- examples of control: feedback, adaptive, integrated control
- overview of conventional control approaches and their
limitations
- motivations for qualitative/model-based reasoning for control
- unit B3.2: QR and medical applications
- how might medical reasoning/tasks benefit from QR
- modeling ontologies for pathophysiological systems
- which level of representation
(pure qualitative, semi-quantitative, quantitative) versus
tasks
examples
Back
to Top
Lecture B4: Diagrammatic Reasoning and
Visualisation of System
Behaviour
(Teacher: Alan Blackwell)
- unit B4.1: introduction and motivation
- what are the problems
- overview on research in this area
- unit B4.2: examples and remaining problems
- problems and solutions (cont'd)
- applications and examples
- future research goals
Back
to Top
Lecture B5: Qualitative Reasoning and Mathematical Modeling
(Teacher: Liliana
Ironi)
- unit B5.1 quantitative modeling
- introduction: what is mathematical modeling, what are the
problems
(physical and numerical accuracy)
- integration of qualitative and quantitative approaches to deal
with:
- automated structural modeling (qualitative analysis of
experimental data, QR for delimiting the search model space, QR
for the choice of proper numerical methods and their possible
initialization)
- non-parametric/black-box modeling (QR for the initialization
of a proper identifier scheme)
- brief overview of qualitative-quantitative integrated approaches
and tools
- unit B5.2 a case study
- Automated modeling of visco-elastic materials and its use for
the assessment of physico-chemical properties of drug-delivery
systems
Back
to Top
Lecture B6: Knowledge Management using MBS/QR Technology
(Teacher: Andy Steele)
- unit B6.1: introduction and motivation
- what are the issues?
(to enable organisations to compete effectively in emerging
knowledge economy; can help represent qualitative/heuristic/tacit
knowledge in easy-to-understand structured format, thus enabling
sharing and re-use of valuable corporate and industrial
knowledge)
- overview on research in this area
(problems and solutions, e.g.: qualitative influence diagrams
for physical or business process modelling)
- unit B6.2: examples and remaining problems
- problems and solutions (cont'd)
- applications and examples (success stories?)
- future research goals
Back
to Top
Lecture B7: Qualitative Spatial Reasoning and Kinematics
(Teacher: Tony
Cohn)
- unit B7.1: introduction, motivation and problems
- what are the problems
- overview on research in this area
- unit B7.2: examples and remaining problems
- problems and solutions (cont'd)
- applications and examples
- future research goals
Back
to Top
Teachers
Teachers were
senior researchers and experienced users with a wide range of insights.
They were able to present clearly the main ideas, point out problems,
discuss outstanding issues, and reflect on the area as a whole. Teachers
were encouraged to stay around during the whole summer school and mingle
with participants, so that they were available for in-depth discussion
and questioning by students.
List of Teachers
Alan
Blackwell
Computer Laboratory
University of
Cambridge
Cambridge, UK
Bert
Bredeweg
Department of Social Science Infomatics
(SWI)
Universiteit van Amsterdam
Amsterdam, The Netherlands
Tony
Cohn
School of Computer Studies
University of
Leeds
Leeds, UK
Luca
Console
Dipartimento di Informatica
Universita' di
Torino
Torino, Italy
Ken
Forbus
Institute for the Learning Sciences
Northwestern
University
Evanston, Illinois, USA
Liliana
Ironi
Istituto di Analisi Numerica (CNR)
Pavia, Italy
Ben
Kuipers
Department of Computer Sciences
The University of
Texas at Austin
Austin, Texas, USA
Andy
Steele
Unilever
London, UK
Peter
Struss
Department of Computer Science
Technical
University of Munich
Munich, Germany
Back
to Top
Location
The Summer School
took place in the University
Residential Centre of Bertinoro.
Bertinoro is half way between Forlė and Cesena, 6 kilometres from
State Road No.9 (Via Emilia), immediately east of Forlimpopoli.
For
details of how to reach the Centre, see the Centre's "How to Reach Us"
page (http://www.spbo.unibo.it/bertinoro/ecomeraggiungerci.html)
You may find the "Ferrovie
dello Stato" (Italian Railway) web site useful for train timetables
etc.
Back
to Top
Organising
Committee
Bert
Bredeweg
Department of Social Science Infomatics
(SWI)
Universiteit van Amsterdam
Amsterdam, The Netherlands
Liliana
Ironi
Istituto di Analisi Numerica (CNR)
Pavia,
Italy
Louise
Trave-Massuyes
LAAS/CNRS
Toulouse, France